A renowned AI researcher, and pioneer, who has made a significant impact in the field of artificial intelligence and deep learning.
For five decades, the contributions of Geoffrey Hinton have been fostering the core technology behind chatbots such as ChatGPT.
He is a leading figure in AI and quit Google on 1st May 2023, Monday, particularly over concerns about the rapid development of AI technologies like ChatGPT.
He has become part of a swelling group of critics who claim that AI companies are wooing danger with their fast-paced creation of products utilizing generative artificial intelligence, the technology that drives well-liked chatbots like ChatGPT.
He believes that these advancements, while having the potential for breakthroughs in many fields, also pose significant risks.
And he now wants to speak openly about the dangers of AI without being tied to any company.
Below in this article let’s discuss some of the contributions of Geoffrey Hinton which also made him referred to as the Godfather of AI
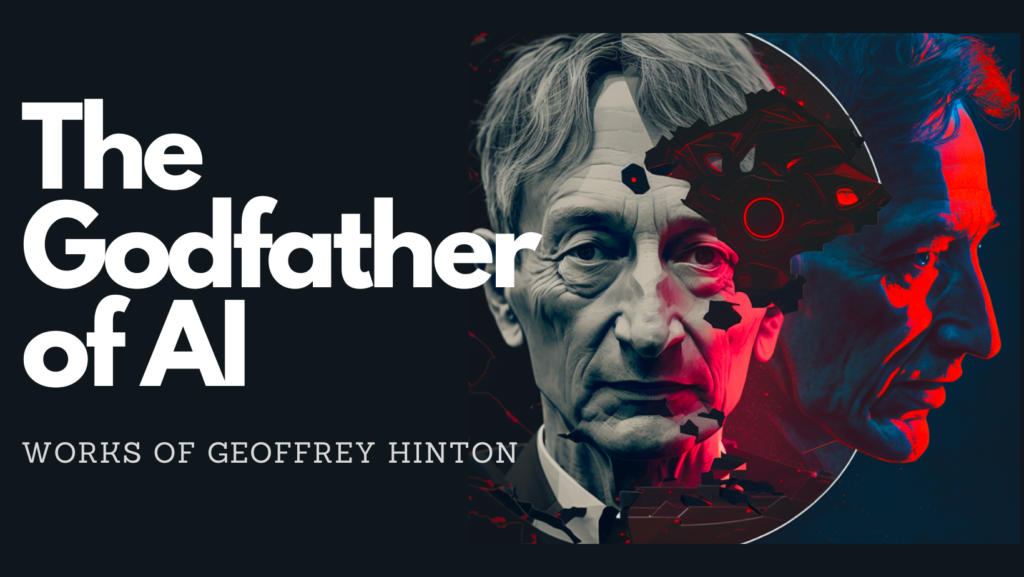
Contributions of Geoffrey Hinton in different branches of AI π
Neural Networks
Hinton developed a number of fundamental techniques for training neural networks, which are computational models inspired by the structure and function of the human brain.
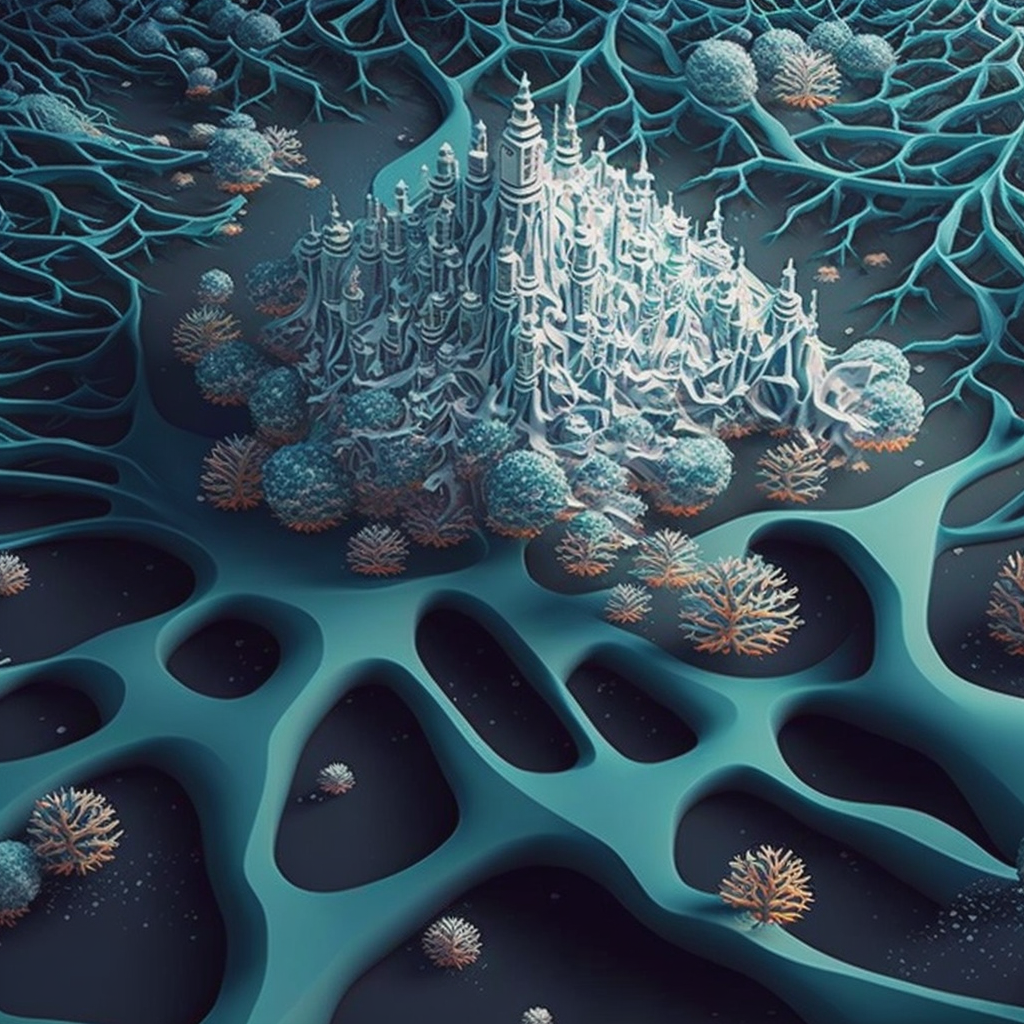

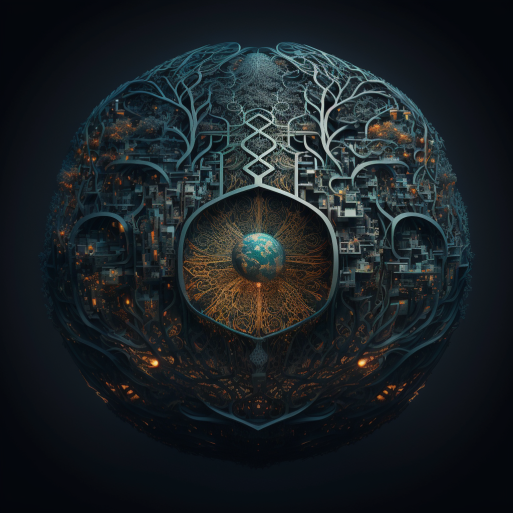
For example, imagine you want to ask a computer a question like “What’s the weather like today?” The computer can use a neural network to figure out the answer. ChatGPT is an example of a neural network that can understand and respond to questions like a human.
- Backpropagation algorithm (1986)
Hinton, along with David Rumelhart and Ronald Williams, developed the backpropagation algorithm in 1986, which is now widely used in deep learning.
Basically, it’s a method for training neural networks that allows the adjustment of the network’s weights to minimize the error between the predicted output and the actual output.
This technique helps computers get better at doing things like recognizing pictures or understanding language. When a computer tries to recognize a picture or understand a sentence, it makes guesses about what it might be. Backpropagation helps the computer figure out what it needs to change in order to make a better guess next time.
Midjourney serves as a prime example of this; take a look at the easy-to-understand article on prompts for beginners to kick-start your creative journey. - Boltzmann machine (1986)
Hinton and Terry Sejnowski developed the Boltzmann machine, which is a type of stochastic recurrent neural network. It uses probabilistic connections between its neurons, which allows it to learn and represent complex patterns in data.
The Boltzmann machine is good at finding patterns that other machines might not be able to see, making it useful for things like speech recognition and image analysis. It’s like having a super smart friend who can help you understand things you might have missed alone! - Contrastive divergence (2002)
Hinton and his collaborators developed the contrastive divergence algorithm, which is now widely used in deep learning.
It’s a learning algorithm for training Boltzmann machines that is faster and easier to use than previous methods.
Suppose you are training a machine learning model to identify pictures of dogs. The model will initially predict that a picture of a cat is a dog, which is wrong. Using the Contrastive Divergence algorithm, the model’s parameters will be adjusted so that it is more likely to predict correctly that the picture is a cat, not a dog.
Deep Learning
Hinton made significant contributions to the development of deep learning, a subfield of machine learning that involves training neural networks with many layers of processing.
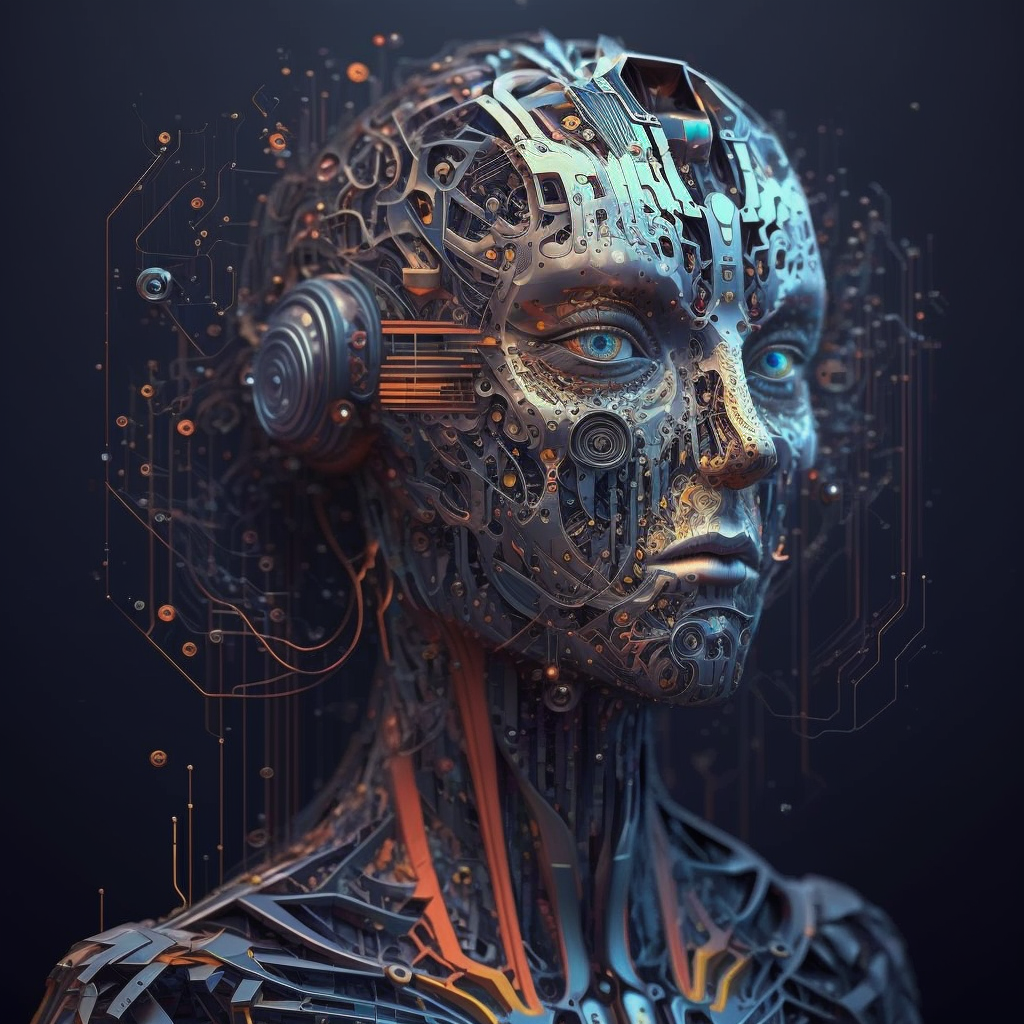
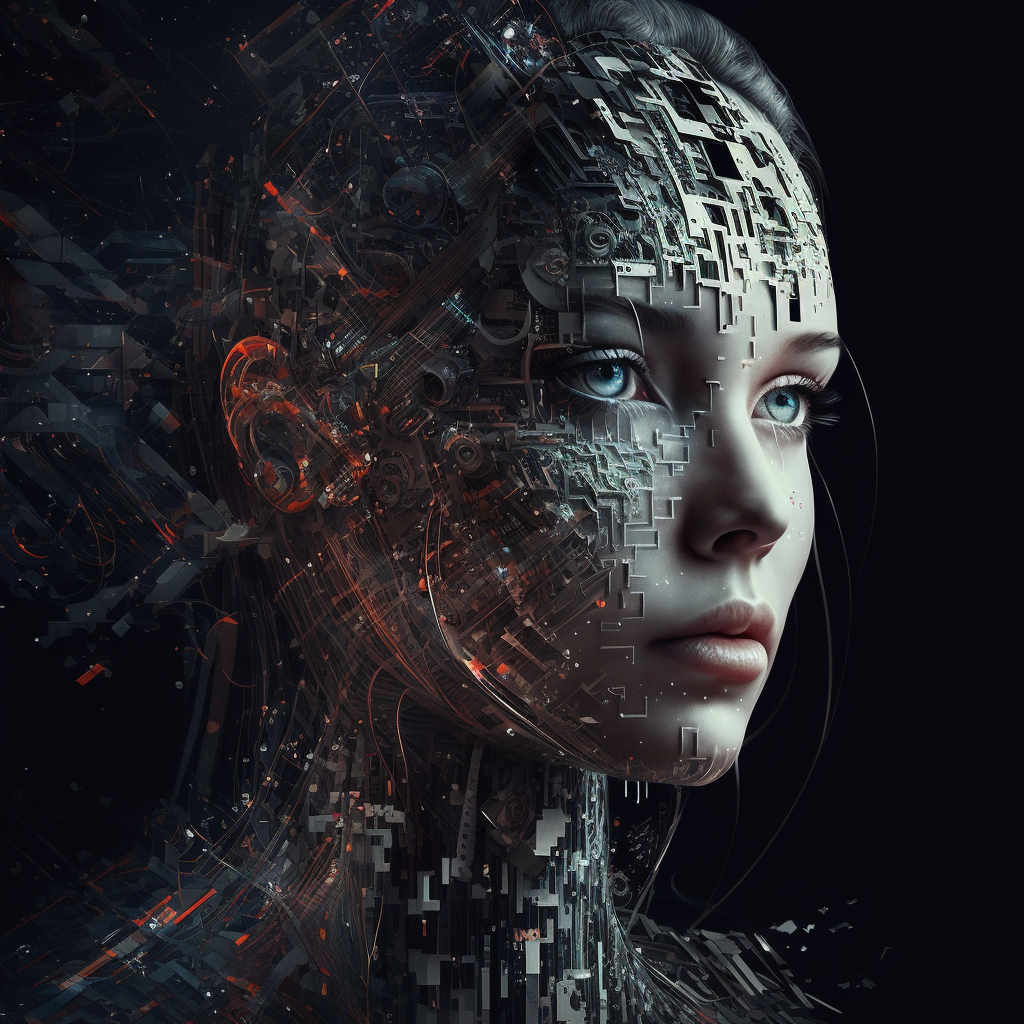
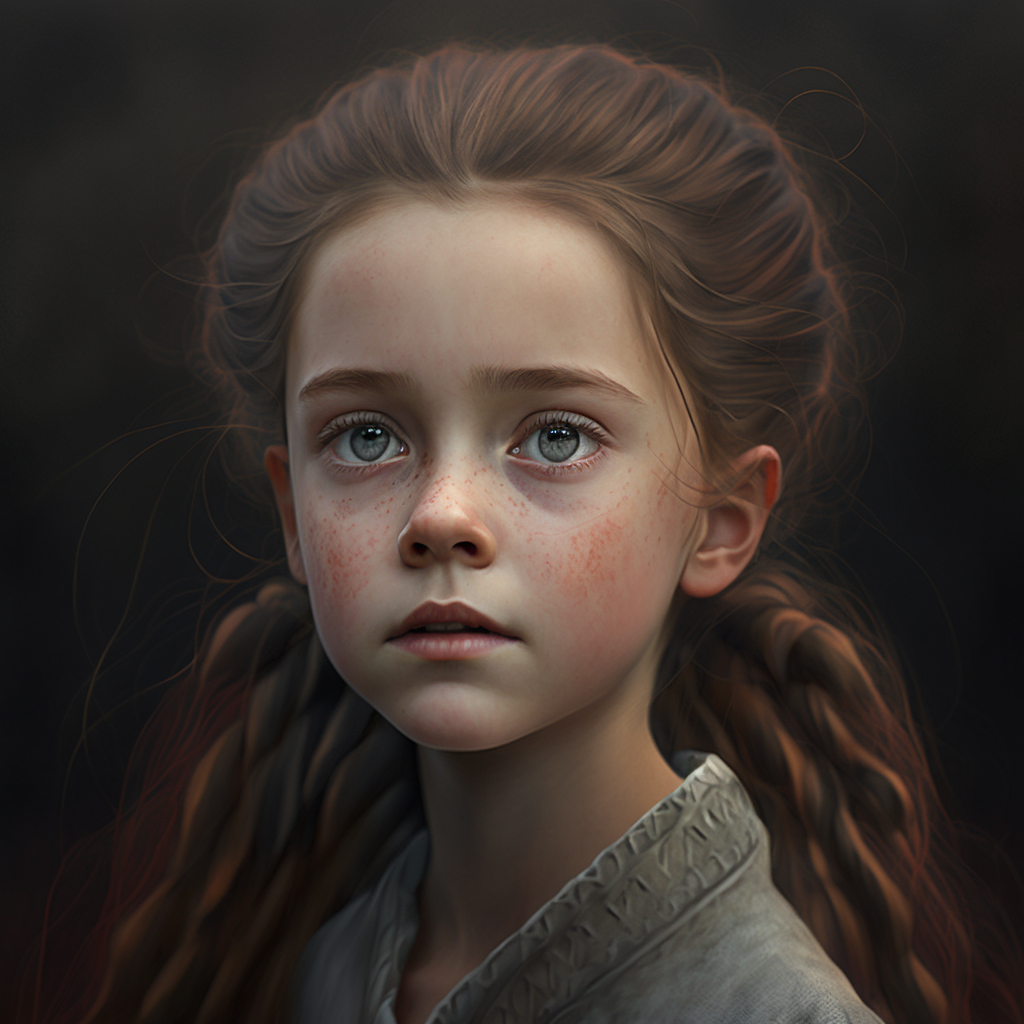
- Deep belief networks (2006)
A type of neural network that can be used for unsupervised learning of complex data.
These networks are often used for tasks like image or speech recognition. One of the key features of deep belief networks is their ability to automatically learn the most important features in the data, without the need for human intervention or feature engineering. - Dropout (2012)
It’s a technique that helps to prevent neural networks from overfitting, which is when they memorize the training data instead of learning to generalize to new data.
Think of it like a student who is trying to memorize a textbook. They might end up memorizing everything, but that doesn’t mean they understand the material. Dropout works by randomly dropping out (ignoring) some of the neurons in a neural network during training, forcing the remaining neurons to work together to learn the data.
Capsule Networks
- Capsule Networks (2017)
Hinton and his colleagues introduced Capsule Networks as an alternative to traditional neural networks.
They are designed to better understand the spatial relationships between objects in an image, which is a limitation of traditional neural networks.
Think of it like a student trying to draw a picture of a house. Instead of just drawing each part of the house separately and then sticking them together, the student tries to draw the house as a 3D object, with all the parts connected in the correct way.
A new type of neural network architecture that allows the network to learn features in a hierarchical manner, which can lead to more accurate and robust predictions.
Hinton and his team further explored Capsule Networks and introduced the concept of dynamic routing. This technique allows capsules to communicate with each other and determine the relationships between objects in an image.
Generative Models
- Generative adversarial networks (2014)
A type of neural network that can be used to generate new data that is similar to a given dataset. Hinton, along with Ian Goodfellow and others, played a key role in the development of GANs.
It’s like a student who learns to draw by showing their drawings to a friend who can tell if it looks real or fake. The student keeps improving their drawings until they are almost as good as the real ones.
In GANs, the student is the generator network, the friend is the discriminator network, and the pictures are the training data. - Neural autoregressive distribution estimator (2016)
A neural network architecture for estimating the probability distribution of a given dataset.
Consider a scenario where you want to predict the likelihood of someone buying a product based on their browsing history on a website. The Neural Autoregressive Distribution Estimator can analyze the user’s behavior and make predictions about which products they are most likely to purchase.
Miscellaneous
- GPT (2018): The Generative Pre-trained Transformer (GPT) is a type of neural network that can generate natural language text. Hinton’s former lab, OpenAI, developed GPT, which has achieved state-of-the-art performance on several natural language processing benchmarks
- Knowledge distillation (2015): A method for transferring knowledge from a large, complex model to a smaller, simpler model, while maintaining most of the accuracy of the larger model.
- Radial basis function network (1988): A type of neural network that can be used for function approximation and classification tasks.
Your post really made me think. I appreciate the new perspectives you’ve introduced here. Keep up the excellent work!
This post was a joy to read. Your enthusiasm for the subject is infectious and makes learning about it fun.